My Account Details
An Introduction to Efficacy Research in Education
Helping schools, colleges and educators make smarter decisions
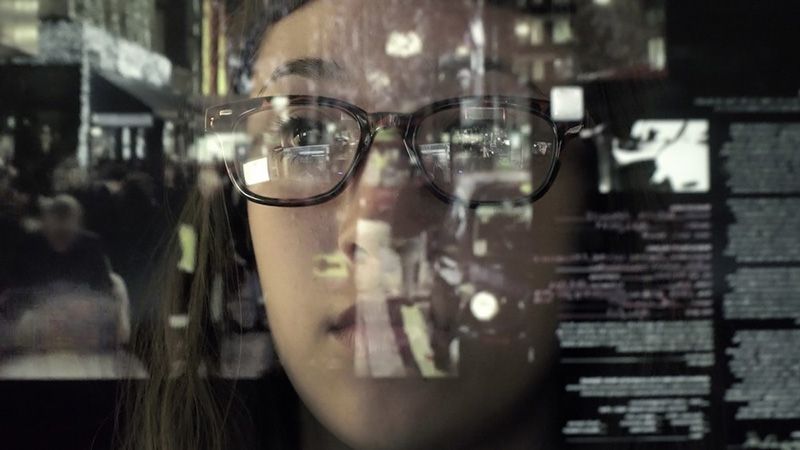
How can we prove that learning technology works? Randomized controlled trials are considered the gold standard in efficacy research, yet they are costly to implement, take years to complete, raise ethical issues, and are difficult to scale in size. Can we democratize the way we look at efficacy in education by leveraging big data and techniques in machine learning, statistics and learning sciences?
In this post, I will explain how we conducted a quasi-experimental study of 3,400 students with minimal cost, time and interference in students' learning. In this study, we applied propensity score matching, a method used to conduct a quasi-experimental study, to demonstrate how ALEKS is helping to improve students' course outcomes [1]. The study was conducted in collaboration with Prof. Ryan Baker at the University of Pennsylvania and published at the International Conference on Learning Analytics and Knowledge. I will also share how we should be thinking about and structuring efficacy research to overcome the limitations of randomized control trials while preserving their rigor.
Why efficacy?
Would a doctor ever prescribe a medicine that hasn't been shown to effectively treat a disease? Would you purchase a car that doesn't pass safety tests?
In healthcare and countless other fields, it's standard for treatments and products to undergo research and testing to validate claims made about their effectiveness. This measure of effectiveness is called "efficacy." or the ability of a product to produce the desired results or effects.
Efficacy research is equally important in education. How can educators and decision-makers at schools and colleges trust that the tools and practices they're using will improve student success? Countless new technologies have been introduced to the education market in the last few decades, all of which claim to improve student learning outcomes in some way or another. This has made it necessary for schools and colleges to closely examine and compare efficacy claims when selecting new learning products.
Add to that the introduction of the Every Student Succeeds Act (ESSA) in 2015. ESSA mandates that the use of federal dollars for school improvement (e.g., Title I, Section 1003) be restricted to programs that show evidence of effectiveness. As a creator and provider of these technologies, it's crucial that McGraw Hill do all it can to accurately measure the efficacy of its products and be transparent about how it conducts its research.
But how exactly are high quality efficacy studies constructed? As a data scientist at McGraw Hill, one of my roles is to think about that important question. How can we design research studies that help us and our customers get a deeper understanding of whether, and under what conditions, our products help students make learning gains?
Traditionally, randomized controlled trials have been considered the gold standard in research design. But new technologies and learning analytics have made it possible to design studies that are less expensive and often more accurate and illuminating. Let me elaborate.
Randomized controlled trials
The traditional format of efficacy research is a randomized controlled trial (RCT). An RCT is an experiment where the study participants are randomly assigned to two groups of treatment and control, where each group receives or does not receive a pre-determined product or instructional technique. Randomization minimizes selection bias and allows the researchers to measure the treatment effects between the groups. This is a research format frequently used in medicine.
Unfortunately, there are some drawbacks to RCTs that are leading education researchers to look at other formats. RCTs are costly and can be tricky to conduct in educational settings. This is due to both the difficulty and ethical concerns of getting consent for randomized assignment, and because of the challenges in maintaining consistency in how different products are used in such trials [2].
Quasi-experimental studies
For these reasons, many researchers are turning to what are called quasi-experimental studies as an alternative to RCTs [3]. These studies are a practical and acceptable alternative to RCTs when implementing a well-designed RCT is implausible [4]. They take less time. They cost less. And they can be completed easily with a much larger sample of participants.
In quasi-experimental studies, participants are sorted into to treatment and control groups based on some criteria, such as their birth date or their score on a placement test, as opposed to in RCTs where this assignment is completely random. The assignment is done through a system known as propensity score matching (PSM) [5][6]. In PSM, for each member of the intervention (treatment) group, we identify a member of the control group (the group that did not receive the intervention) that is as similar as possible to a member of the treatment group based on a set of those factors, like placement test scores. Therefore, with PSM we can factor out many common extraneous differences between students that can have an effect on their performance before we compare their outcomes. Then, the difference in outcomes between the matched pair is computed. The average of this difference over many observed pairs is an estimate of the average impact of a particular intervention on learning outcomes. Importantly, PSM can be done post-hoc. We can ask a group of students to participate in a study and use a McGraw Hill product, and then find a matching set of students from a large general population of non-users.
Here's how we've done it at McGraw Hill:
I recently led a study evaluating the efficacy of ALEKS for students taking college-level math classes within the context of a large Midwestern community college. Data was collected from 3,400 students in 198 sections covering four courses, including pre-algebra, elementary and intermediate algebra, and college math. In this study, students were not randomly assigned into their classes, so doing a quasi-experimental study made sense, as PSM allows us to create comparable treatment and control groups.
As is often the case in education, there is inconsistency in how different instructors used the technology with their students. In this college, some class sections volunteered to use ALEKS while others did not. Within ALEKS classes, however, only a portion of students ended up using ALEKS, despite the recommendations of the instructors.
Basically, we recognize that some students who are in classrooms where ALEKS is assigned still did not use the program. The college told us that those students were likely the most unmotivated students to begin with, so they might show poor scores in the course for reasons other than the effectiveness of the technology. So we designed the quasi-experimental study by matching students who we knew did use ALEKS against students who were in the non-ALEKS classrooms and didn't have a choice. We used propensity score matching (PSM) along with factors like birth date and placement test score to find ideal student-to-student matches. In the final design of the study, we matched ALEKS students to non-ALEKS students in non-ALEKS sections to be able to conduct a fair comparison between the two groups. In the end, we found that students using ALEKS achieved significantly higher pass rates than comparison group. When matching students using PSM, students who use ALEKS passed 15 percentage points compared to non-ALEKS students. This means that students using ALEKS are 1.27 times more likely to pass the course than students not using ALEKS.
Research is at the foundation of our mission to help all learners succeed. As the educational technology market continues to evolve, we'll continue to turn to real classrooms to find new ways to evaluate and demonstrate the impact of our digital learning tools.
References
[1] S. Mojarad, A. Essa, S. Mojarad, and R. S. Baker, "Studying Adaptive Learning Efficacy using Propensity Score Matching."
[2] M. Feng, J. Roschelle, N. Heffernan, J. Fairman, and R. Murphy, "Implementation of an intelligent tutoring system for online homework support in an efficacy trial," Lect. Notes Comput. Sci. (including Subser. Lect. Notes Artif. Intell. Lect. Notes Bioinformatics), vol. 8474 LNCS, no. 2013, pp. 561–566, 2014.
[3] D. Kaplan, "Causal Inference in Educational Policy Research."
[4] G. M. Sullivan, "Getting Off the 'Gold Standard': Randomized Controlled Trials and Education Research," J. Grad. Med. Educ., vol. 3, no. 3, pp. 285–289, Sep. 2011.
[5] P. C. Austin, "An Introduction to Propensity Score Methods for Reducing the Effects of Confounding in Observational Studies," Multivariate Behav. Res., vol. 46, no. 3, pp. 399–424, May 2011.
[6] P. R. ROSENBAUM and D. B. RUBIN, "The central role of the propensity score in observational studies for causal effects," Biometrika, vol. 70, no. 1, pp. 41–55, Apr. 1983.