The Growing Resource Demands of AI and Potential Solutions
The Energy Footprint of AI: A Growing Concern
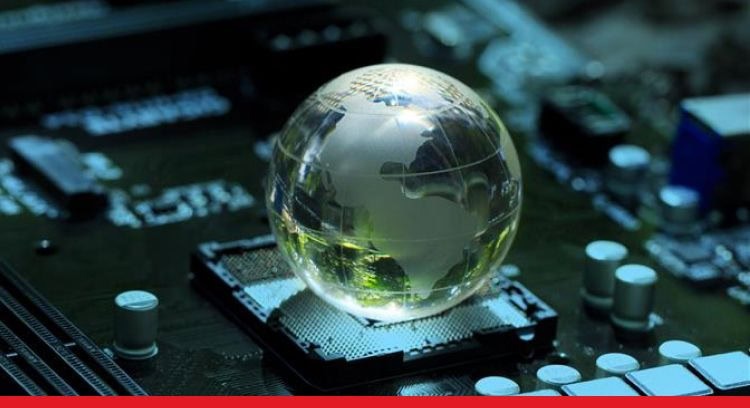
As artificial intelligence (AI) continues to evolve and integrate into various sectors, its energy requirements are becoming a significant concern. The rapid expansion of AI, particularly through large language models (LLMs), such as GPT-4, and other emerging platforms, is driving up the demand for data centers, which are notoriously energy-intensive. This post explores the projected energy requirements associated with AI growth, the impact on other natural resources, such as water, and potential solutions to mitigate AI’s environmental impact.
The Surge in AI Adoption
The adoption of AI has skyrocketed in recent years. According to a McKinsey Global Survey, the use of generative AI nearly doubled from 2023-2024, and this trend is expected to continue, with an annual growth rate of over 40% for the next decade.
AI is also being adopted into advanced educational tools. More recently, a 60 Minutes feature investigated Khanmigo, an AI-powered tutor, as a way to help both educators and students. AI can significantly enhance the efficiency of educational processes and provide personalized learning experiences, which are key trends in education.
However, the speed at which AI adoption is occurring has consequences, particularly in terms of energy and water consumption.
Energy Demands of Data Centers
Data centers, the backbone of AI operations, are experiencing a significant increase in energy demands. McKinsey predicts that global demand for data center capacity could rise by as much as 22% annually for the rest of the decade while the International Energy Agency (IEA) estimates that global data center energy demand could double by 2026. Research from Goldman Sachs predicted data centers will use 8% of US power by 2030, compared with 3% in 2022.
As data centers come online, they will use a considerable amount of energy. In fact, McKinsey estimates that energy demands from data centers could be as high as 298 gigawatts by 2030, the equivalent of the amount needed to power more than 200 million residential homes. The growing energy needs of AI are also putting pressure on electrical grids.
These energy increases are not just due to the sheer volume of data processed but also the intensive cooling systems required to maintain optimal operating temperatures.
Impact on Water Resources
In addition to energy, AI’s growth significantly impacts water resources. Data centers require vast amounts of water for cooling purposes. For instance, AI operations could necessitate water withdrawals of 4.2 to 6.6 billion cubic meters by 2027. This is more than the total annual water withdrawal of several small countries combined.
The water footprint of AI is substantial because it involves both direct and indirect consumption. Directly, water is used to cool the servers and maintain optimal operating conditions. Indirectly, water is consumed in the production of the hardware required for AI, such as semiconductors and microchips.
In summary, the environmental impact of AI’s resource consumption cannot be ignored. The carbon footprint of data centers is substantial, and without significant changes, the growth of AI could exacerbate climate change and lead to water shortages and affect local communities.
Strategies for Reducing AI’s Energy and Water Footprint
- Energy Efficiency Improvements and Geographical Distribution: Optimize server utilization, improve cooling systems, adopt energy-efficient hardware, and strategically locate data centers in regions with abundant renewable energy and sufficient water supplies to reduce strain on local resources.
- Renewable and Nuclear Energy Integration: Increase the use of renewable energy sources and integrate nuclear power. For example, Microsoft has committed to purchasing energy from the Three Mile Island nuclear reactor, providing a stable and low-carbon energy source. Across the tech sector, companies like Microsoft, Google, Amazon and Meta are all getting into nuclear tech.
- AI-Driven Optimization: Utilize AI to enhance energy efficiency, and resource management. AI can optimize data center operations, predict maintenance needs, and improve cooling systems, reducing overall energy and water usage.
- Innovative Cooling Solutions: Implement advanced cooling technologies, such as liquid immersion cooling, direct-to-chip cooling, geothermal cooling (e.g. using earth’s stable underground temperatures) and natural cooling sources (e.g. building data centers in cooler climates), to enhance efficiency.
- Policy and Regulation: Governments can set standards for energy and water efficiency, incentivize renewable and nuclear energy use, and support green data center development.
The Future of AI’s Footprint
The growth of AI presents both opportunities and challenges. While the increasing energy and water demands are a concern, there are viable solutions to mitigate their impact. By focusing on energy and water efficiency, renewable energy integration, strategic data center placement, innovative cooling solutions, and supportive policies, we can ensure that the advancement of AI aligns with our environmental goals. The future of AI and resource sustainability depends on our collective efforts to innovate and adapt.
Reflection Points
- What are your thoughts on the balance between AI advancement and resource sustainability?
- Would you be willing to consider the construction of additional nuclear power plants or small nuclear reactors near your community? Why or why not?
- Would you be willing to use less water or energy in order to have access to AI?
- Will the social and environmental contributions of AI be overshadowed by its huge energy water footprint in the longer term? Why or why not?