My Account Details
What is Adaptive Learning Anyway?
A look at the science and research behind adaptive learning technology and its application in the classroom.
This post originally appeared on LinkedIn Pulse on January 5, 2017 and can be viewed here.
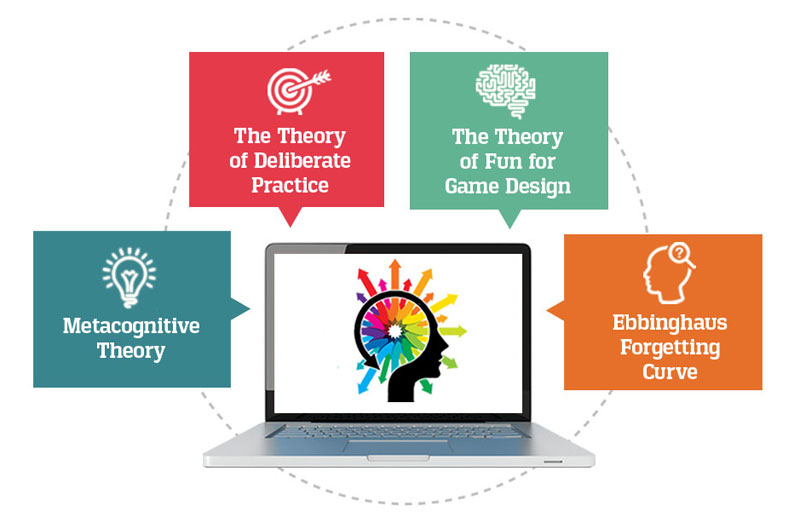
As emerging technologies and methodologies reshape corporate training, one phrase “adaptive learning” remains the biggest of the buzzwords.
I get asked all the time what exactly “adaptive learning” means. Most know the technology has something to do with e-learning, personalized education, micro-learning, badging, gamification, cloud-based learning, etc. But what does it really mean? How feasible is it to implement? And where can you get your hands on it?
Imagine that you could give every learner their own personalized course, made specifically for their strengths, weaknesses, goals, and engagement patterns. Imagine a course that adapted in real-time to their activity and adjusted moment by moment to their performance and interest level.
That’s adaptive learning.
How adaptive learning works
To make this learner-centered vision of education a reality, the field uses artificial intelligence to actively tailor content to each individual’s needs. It draws upon knowledge domains as diverse as machine learning, cognitive science, predictive analytics and educational theory.
For example, the visual below on the left shows individuals moving through content in a conventional, linear setting. Every learner must encounter every piece of content. On the right, a learner is experiencing only the content that she needs to see at any given moment, given her mastery of the subject.
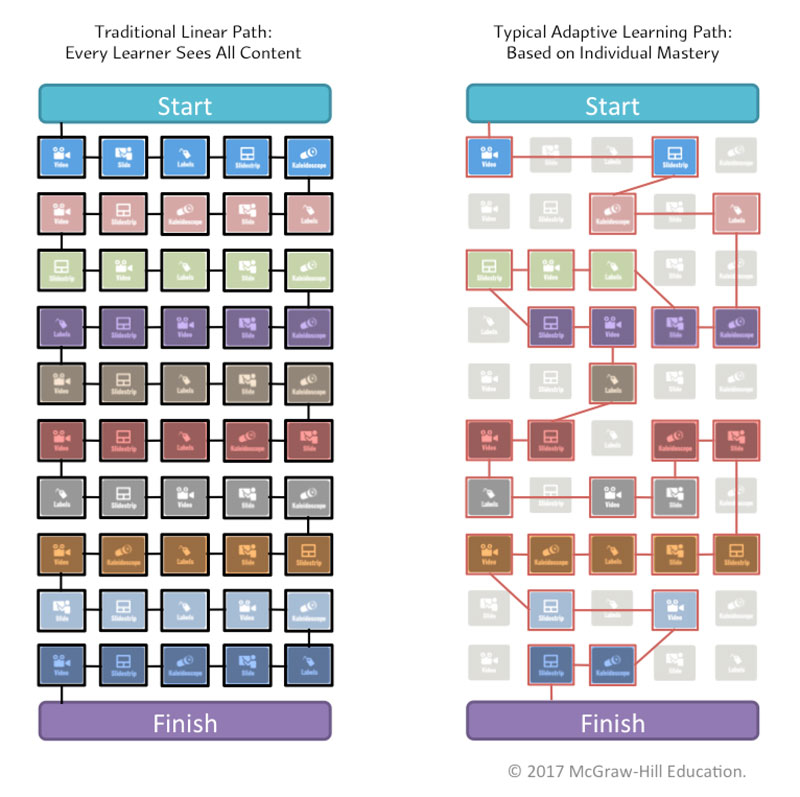
Some distinctions can help clarify the concept. “Adaptive learning,” while related to “adaptive testing,” has a completely different goal. Both “adaptive learning” and “adaptive testing” adjust the content based on learner activity and performance. However, the aim of “adaptive testing” is to figure out each learner’s proficiency or skill-level in as few questions as possible (hence the GRE or GMAT). The goal of “adaptive learning” is to help each learner learn as efficiently and effectively as possible. When I say “learn,” I mean, gain demonstrable proficiency in some learning objective, to experience an ah-ha moment.
Traditional learning vs. Mastery-based instruction
Since the Industrial Revolution, learning has generally fit the lecture-based, one-size-fits-all model. In this model, a few learners in any cohort excel, but many simply pass without having mastered important concepts. Many learners get Cs and perhaps a few get As, while a few fail. This reality has simply been accepted for most of history, hence the typical grade distribution curve we are all familiar with. There are other characteristics of traditional learning which have also gone largely unexamined:
- the idea of grouping learners together based on their age rather than skill level
- the lack of integration between formal education and informal (as well as experiential learning)
- an emphasis on summative rather than formative assessment—think high-stakes, nerve-wracking final exams as opposed to quick-checks for understanding and opportunities for reflection
The list goes on and on.
By contrast, mastery-based instruction places the emphasis on mastery rather than seat-time, which generally leads to higher proficiency and engagement levels for all learners. For those who are unfamiliar with the concept, “mastery-based” learning has a few basic tenets:
- Progression through a course of study should be based on proficiency rather than hours spent.
- Learners cannot give up.
- Learners must achieve proficiency in order to progress and complete the course.
- Learners can spend however long they need to master concepts.
The thinking is fundamental to a more learner-centric model of education.
Of course one-on-one mentorship with an instructor who understands each learner’s strengths weaknesses, unique background and learning preference is what typically leads to the highest levels of mastery. Many consider this to be the ideal, but private tutors are expensive and generally reserved for only a few subject areas like high-stakes testing. Most learners never receive this kind of attention and thus, never achieve the mastery levels they might have otherwise.
None of this thinking is new. In 1984 educational psychologist Benjamin Bloom reported in his famous 2 Sigma Problem paper, published initially in Educational Researcher, that the average learner in a one-to-one mastery-based learning situation performed two standard deviations better than the average learner in a conventional setting. What that means is that 98% of the learners in the one-to-one mastery-based situation performed as well as the average learner in the traditional setting.
Making adaptive learning a reality
What’s exciting about our times is that we now have technology to make these ideas real and scalable and, in the process, deliver dramatically better learning outcomes. Adaptive learning technology inexpensively scales the benefits of one-on-one mentorship, providing each learner with their own personalized course, which adjusts in real-time for his or her performance and engagement level.
At McGraw Hill, we unlock performance by driving mastery and measurable results through a powerful data layer. When our clients' content is delivered on our platform, it creates a data layer that powers not only adaptive learning but also tailored instruction and agile authoring. The benefits are summarized below:
Learners receive the right content at the right time, maximizing learning efficiency, effectiveness, engagement, and retention.
Instructors/Trainers/Managers grasp cohort dynamics through real-time analytics and know exactly what their learners are struggling with, so they can quickly adapt instruction.
Content authors understand what content works and doesn’t work, so they can continually refine content.
The technology is based on educational theory and cognitive science that explores intuitive design, metacognition, memory and the personalized delivery of concepts. Over the past decade, McGraw Hill has invested over $500 million (via organic growth and strategic acquisitions) to make this vision of scientifically based learning a reality.