My Account Details
How Artificial Intelligence Can Help Us Solve the 33-Year-Old “Two-Sigma Problem”
Artificial Intelligence is here, offering every student to reach their full potential in the classroom.
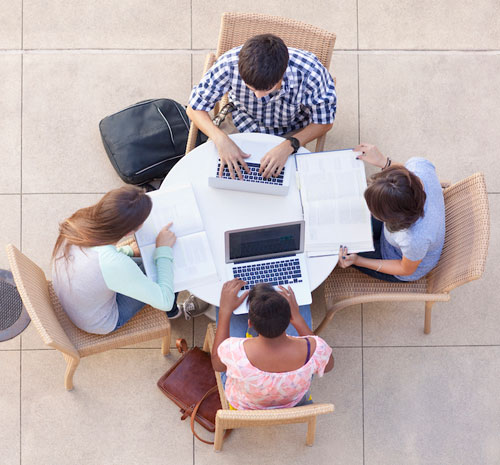
We used to talk about artificial intelligence (AI) as a “new frontier” for technology – something exciting and powerful that will inevitably affect our lives but is still a ways down the road.
That’s no longer the case. AI is here and we’re interacting with it every day, from virtual assistants like Siri and Alexa to personalized user experiences on Amazon and Netflix. It is changing how students learn as well. AI allows educators to deliver the kind of learning experiences that researchers have long understood to be most impactful – namely, “mastery learning” environments supported by one-to-one tutoring – but that in the past were just not scalable.
Frankly, it’s precisely the kind of technology educators have been waiting for more than three decades – since a seminal piece of research hinted at just how powerful these approaches might be.
In the mid-1980s, an educational psychologist named Benjamin Bloom, best known for his taxonomy of learning domains, set out to answer some seemingly simple questions: Are there concrete adjustments we can make to the classroom format – say, giving every student a personal tutor or focusing on mastery learning – that can have a positive impact on student performance? If so, how great of an impact can each of those adjustments have? And are any scalable?
By approaching his inquiries experientially, Bloom – who passed away 18 years ago this month – uncovered groundbreaking findings on learning and teaching, and advanced the then-nascent field of learning science immeasurably.
The Two-Sigma Problem
So just what did Bloom discover, and how did he do it?
Bloom’s research evaluated groups of students learning under three different ways of teaching:
- The first group was placed in a conventional “sage on a stage” model of one teacher lecturing to a group of roughly 30 students. This approach resulted in the standard bell-shaped curve of student performance – lots of students in the middle and some at each end.
- The second group took the same ‘sage on a stage’ model, but supplemented it with a mastery-based teaching approach. This required that every student demonstrate mastery of each step of a lesson before moving on to the next.
- For the third group, Bloom took the mastery learning approach, and added one-on-one tutoring for each student.
The results were striking: The mastery learning approach alone helped to improve student performance by more than a full standard deviation – representing an average improvement of about one full letter grade. The students who had received personal tutoring in addition to mastery learning did even better: The “average” student in the mastery-plus-tutoring environment performed two full standard deviations better than the average student in the traditional group – mastery learning, in conjunction with personalized tutoring, had produced a “two-sigma” effect on performance.
The major takeaway: The notion of categorizing students as “high” or “low” achievers was almost entirely incorrect. Students who perform in the 50th percentile in a traditional classroom could effectively perform in the 98th percentile with mastery learning plus one-on-one support.
Supporting Mastery at Scale
Now, given that Bloom’s findings were so overwhelmingly positive, why did he declare them to represent a “two-sigma problem”? Simply put, adjustments that had caused the two-sigma effect were not scalable – since it’s often impractical to wait for every student to achieve mastery in one lesson before moving on to the next, and virtually no school has the resources to assign a full-time tutor to every student. Instead, Bloom set about trying to find other ways to bridge the two-sigma gap through other findings from his research.
Almost immediately, educators began adopting various elements of the mastery learning approach wherever possible. For about two decades, that seemed like the best we could hope for – a moderately widespread use of mastery learning principles, perhaps supported by some tutoring when resources allowed.
In the last decade, artificial intelligence and adaptive technology have matured, making both mastery learning and one-on-one instructional moments more scalable than Bloom ever could have imagined.
When it comes to facilitating continuous, personalized mastery learning, modern AI is almost perfectly suited to the task. Using formative assessment – essentially allowing learning by failure with low stakes – AI adaptive learning tools can track precisely how a student is progressing, and then harness those insights to serve up learning experiences designed specifically to help that particular student. By drawing upon a variety of well-established learning science principles, these tools continuously support each student on his or her path toward mastery.
At the same time, by partially automating processes that fundamentally support each student’s mastery learning at his or her own pace, these tools take routine, administrative work away from the instructor freeing up time for higher value interactions with the student. And by informing teachers about exactly how students are doing and where they need more attention, AI facilitates more regular and more targeted “tutor” moments between classroom teachers and their students – making use of that freed up time.
It is a wonderful dream to offer a one-to-one tutor to everybody. But modern AI can now truly replicate the standards that are intrinsic to mastery-based learning, and at the same time offer insights that support tutoring. AI now gives us the opportunity to achieve the meaningfully personalized classroom experiences that Bloom determined to be most effective more than 30 years ago.
If he were alive today, I imagine he would find this very exciting. His legacy lives on.
This article was originally published on HuffPost and can be viewed here.