My Account Details
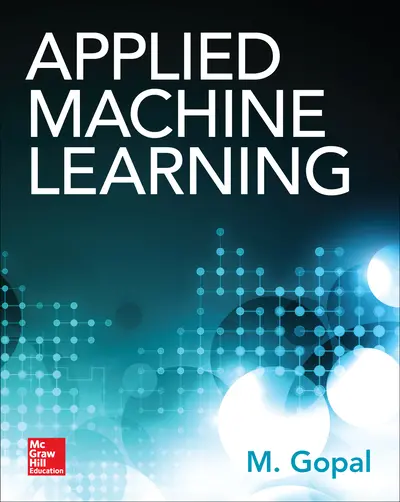
ISBN10: 1260456846 | ISBN13: 9781260456844
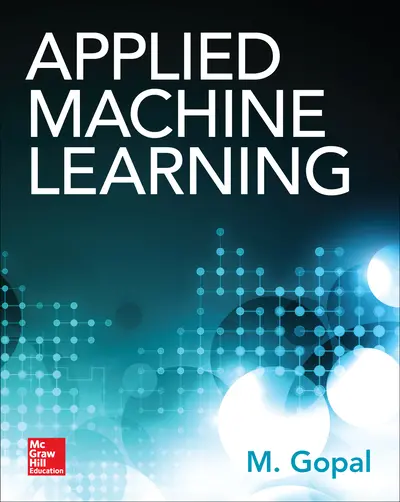
* The estimated amount of time this product will be on the market is based on a number of factors, including faculty input to instructional design and the prior revision cycle and updates to academic research-which typically results in a revision cycle ranging from every two to four years for this product. Pricing subject to change at any time.
Instructor Information
Quick Actions (Only for Validated Instructor Accounts):
Need support? We're here to help - Get real-world support and resources every step of the way.