My Account Details
What Data Reveals About the Connection Between Confidence and Learning Success
Academic work requires students to solve complex tasks demonstrating skills that range from simple to ones that have interdependencies of cognitive, emotional, and social abilities.
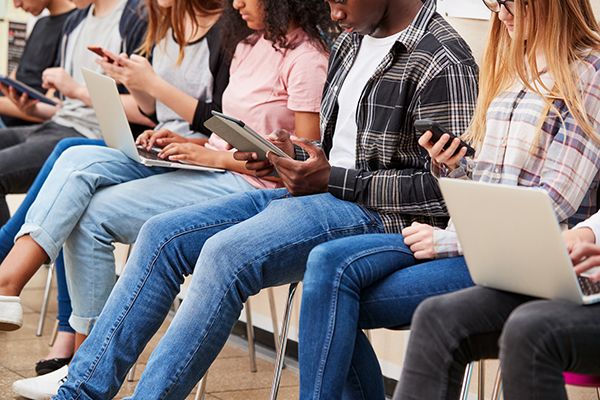
Academic work requires students to solve complex tasks demonstrating skills that range from simple to ones that have interdependencies of cognitive, emotional, and social abilities. The widespread use of online learning environments adds the expectation that students demonstrate knowledge while effectively managing their learning, using study time strategically, and monitoring their own performance. Their success depends on the ability to accomplish these tasks using cognitive and metacognitive skills.
While cognitive skills have long been a primary focus of education, metacognitive skills are increasingly being considered 21st century competencies that are crucial for success. Despite calls for richer learning throughout the history of education, educational practice has been focused mostly on knowledge, cognition, and comprehension. This trend has changed in recent years as technological advancements have made it possible to collect large-scale data on a broader set of skills and competencies and show their effects on success.
In fact, there is already a plethora of research (Barrick, Mount, & Judge, 2003; Poropat, 2009) showing the important role these additional factors play in successful learning. Our interest in confidence (i.e., one’s belief in oneself and capabilities) is part of this larger investigation into the impact of these metacognitive factors on learning outcomes. We believe that confidence, along with other personal characteristics, contributes to a broader picture of the learners’ abilities and their academic behavior. In our research we focus on how students’ confidence interacts with their performance in class, how it changes throughout the course period, and how it varies from discipline to discipline. This kind of information has the potential to yield important insights on how to help students learn to regulate their confidence.
Research suggests that students are not always aware of what they know, and their performance related expectations are not necessarily realistic. Some students get overconfident, and think they are going to succeed when they don’t actually know what they are doing. Other students get anxious, become underconfident, and don’t realize how much they actually do know. However, in either case it is crucial to support their confidence since research suggests that students succeed academically only if they want to and feel capable of doing so (Stiggins, 1999). But how do the dynamics of confidence play out in an actual course using an online learning environment?
To understand this better, we conducted the largest study of student confidence ever -- in the context of McGraw-Hill’s SmartBook platform within Connect. Students in the platform work through practice assignments in their digital course materials, and after every question they rate their own confidence as part of learning how to better know what they know. We obtained this natural data for over 60 million responses by over 129,000 anonymous undergraduate students, and then we compared each student’s confidence to their actual answers. This work was published in the proceedings of the Seventh and Eighth International Learning Analytics & Knowledge Conferences (Aghababyan, Lewkow, & Baker, 2017; Aghababyan, Lewkow, Burns, & Suarez-Garcia, 2018).
In this work, we looked at confidence in terms of four profiles depicted in a 2x2 table:
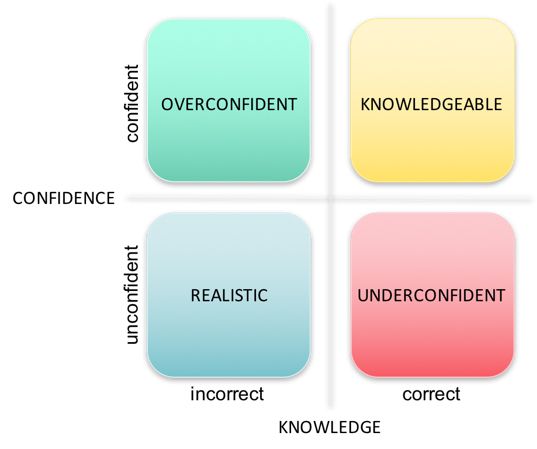
Importantly, we did not just treat confidence as a static measure but we looked at how it changed over time. We also looked into the correlation between students’ proportion of overconfidence and underconfidence and their average score in the adaptive course materials.
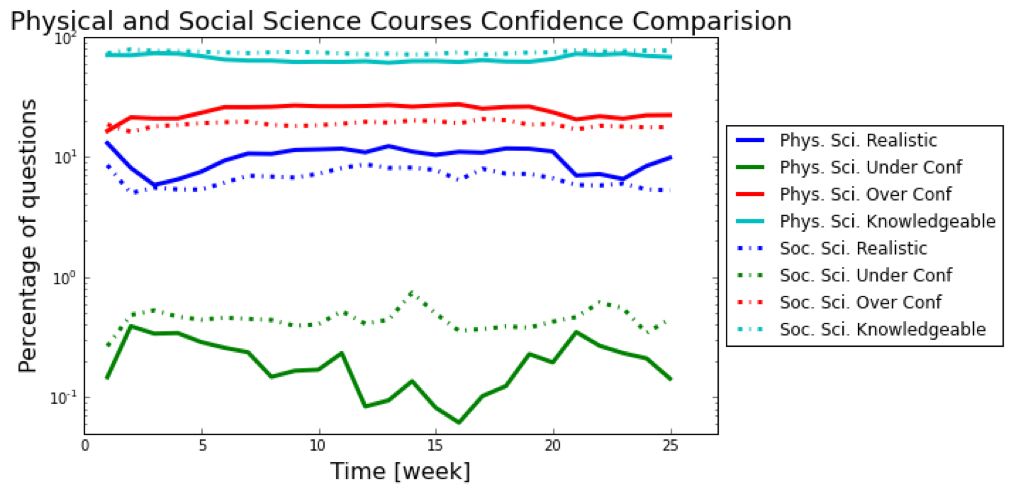
Below are some of the key takeaways from our study:
- Students’ perception of their abilities is asymmetric: they are rarely underconfident (under 1% of responses), but they are often overconfident in their knowledge (almost 20% of responses).
- Students’ perception of their confidence is correlated with their actual performance. However, more successful students also tend to be more overconfident. Overconfidence is not something that happens for the worst students, it’s something that happens for the best students.
- Students tend to be significantly more overconfident when taking courses in the physical sciences than the social sciences. However, stronger students are more likely to be overconfident in the social sciences than in the physical sciences.
- While students’ confidence varies throughout courses, there is more variance in confidence in the first and last weeks of a course.
- Men are more likely to be overconfident than women, but both men and women adjust their confidence similarly based on feedback that they are wrong.
- Overall, students’ perception of their confidence is correlated with their actual performance.
These findings suggest that different students -- and students in different contexts -- need different amounts of support in developing appropriate levels of confidence. By understanding where overconfidence (and underconfidence) are particularly prevalent, we can determine if existing learning systems are inadvertently supporting some students less effectively than others.
These findings then feeds into our work to develop products which better differentiate instruction for different learners. In specific, we are building this research into our efforts to more accurately measure the degree to which a student’s confidence is stably too high or too low. Overall, by recognizing whether an individual student’s confidence is too high, too low, or just right -- we can drive learning supports that help students better know what they know.
References
Aghababyan, A., Lewkow, N., & Baker, R. (2017, March). Exploring the asymmetry of metacognition. In Proceedings of the Seventh International Learning Analytics & Knowledge Conference (pp. 115-119). ACM.
Aghababyan, A., Lewkow, N., Burns, S., & Suarez Garcia, J. (2018, March). Gender differences in confidence reports and in reactions to negative feedback within adaptive learning platforms. Poster presented at 8th International Conference on Learning Analytics & Knowledge. Sydney, Australia: ACM.
Barrick, M. R., Mount, M. K., & Judge, T. A. (2003). Personality and Performance at the Beginning of the New Millennium: What Do We Know and Where Do We Go Next? International Journal of Selection and Assessment, 9(1‐2), 9–30. https://doi.org/10.1111/1468-2389.00160
Poropat, A. E. (2009). A meta-analysis of the five-factor model of personality and academic performance. Psychological Bulletin, 135(2), 322–338. https://doi.org/10.1037/a0014996
Stiggins, R. J. (1999). Assessment, student confidence, and school success. The Phi Delta Kappan, 81(3), 191-198.
Ani Aghababyan, Ph.D.
As a Sr. Data & Learning Scientist at McGraw-Hill, Ani is the lead researcher on the design and the development of an adaptive learning algorithm, while simultaneously evaluating the learning science implications of the product. In her work, Ani uses large-scale educational data to observe and improve student learning trajectories within digital learning solutions. In particular, her research is concentrated on the metacognitive factors of learning that may influence students’ performance and help find optimal learning pathways to better outcomes. As part of her goal to improve student results in McGraw-Hill products, Ani has been publishing the result of her research with her colleagues at partnering Universities.
Dr. Aghababyan is also a lecturer at Northeastern University in their Master of Professional Studies in Analytics program where she teaches statistics & machine learning using R. Additionally, she has been serving as a chair for Doctoral Consortium and Practitioner Tracks for Learning Analytics and Knowledge conference. Ani Aghababyan holds an M.Sc. in Information Systems, an MBA, and a Ph.D. in Instructional Technology & Learning Sciences.
Ryan Baker, Ph.D.
Ryan Baker is Associate Professor at the University of Pennsylvania, and Director of the Penn Center for Learning Analytics. His lab conducts research on engagement and robust learning within online and blended learning, seeking to find actionable indicators that can be used today but which predict future student outcomes. Baker has developed models that can automatically detect student engagement in over a dozen online learning environments, and has led the development of an observational protocol and app for field observation of student engagement that has been used by over 150 researchers in 4 countries. Predictive analytics models he helped develop have been used to benefit hundreds of thousands of students, over a hundred thousand people have taken MOOCs he ran, and he has coordinated longitudinal studies that spanned over a decade.
He was the founding president of the International Educational Data Mining Society, is currently serving as Editor of the journal Computer-Based Learning in Context, is Associate Editor of two journals, was the first technical director of the Pittsburgh Science of Learning Center DataShop, and currently serves as Co-Director of the MOOC Replication Framework (MORF). Baker has co-authored published papers with over 300 colleagues.